Tsinghua University announced that the team of Professor Huaqiang Wu and Associate Professor Bin Gao from the School of Integrated Circuits at Tsinghua University has developed the world’s first fully system-integrated amnesia memory-computing chip that supports highly efficient on-chip learning (machine learning that can be accomplished directly on the hardware side) based on the memory-computing computing paradigm. The results have been published in the latest issue of Science.
According to the article, the team of Qian He and Wu Huaqiang has been on a long march for 11 years, from amnesia devices to prototype chips to system integration, to solve the bottleneck problem of AI arithmetic, and to overcome the “neck” of the key core technologies, and the results involve the amnesia integrated chip, the integrated system, the accelerator of ADAM algorithm, and the system of storage and calculation. system, ADAM algorithm gas pedal …… It is expected to promote the development of artificial intelligence, automatic driving, wearable devices and other fields.
It is understood that in 2012, Qian He and Wu Huaqiang’s team began to study the use of memristors for storage. Memristor is the fourth basic component of circuit after resistor, capacitor and inductor. It can still “remember” the passing charge after power failure, so it is used as a new type of nanoelectronic synaptic device. 2020, the team built a complete storage and calculation system based on multi-array amnesia with all hardware components, which efficiently operated the convolutional neural network algorithm, and successfully verified the image recognition function, which is two orders of magnitude more energy-efficient than the graphic processor chip. The system runs the convolutional neural network algorithm efficiently and successfully verifies the image recognition function, which is two orders of magnitude more energy-efficient than the graphic processor chip, dramatically increasing the computing power of the computing device and realizing the completion of complex computation with smaller power consumption and lower hardware cost.
On-chip learning is important for edge smart devices to adapt to different application scenarios. Current techniques for training neural networks require moving large amounts of data between computing and storage units, which hinders the realization of learning on edge devices.
In this research, Qian He and Huaqiang Wu led a team to innovate and design a new generalized algorithm and architecture STELLAR for efficient on-chip learning applicable to amnesia storage-computing integration, which includes its learning algorithms, hardware implementation, and parallel conductance tuning scheme, and is a generalized approach to facilitate on-chip learning through the use of amnesia cross-gate arrays.
The chip is known to perform tasks such as motion control, image classification and speech recognition. It consumes only 1/35th of the power consumption of an ASIC for the same task, while promising a 75-fold improvement in energy efficiency.
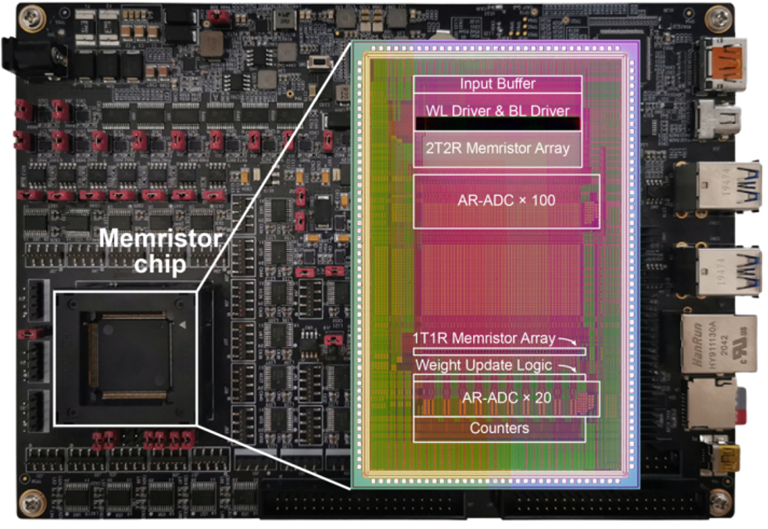
“The integrated on-chip learning can effectively protect user privacy and data while realizing lower latency and smaller energy consumption.” Yao Peng, one of the first authors of the academic paper and a postdoctoral fellow, introduced that the chip can realize fast “on-chip training” and “on-chip recognition” of different tasks with reference to the bionic brain-like processing method, which can effectively complete the incremental learning tasks in edge computing scenarios and adapt to new scenarios and learn new knowledge to meet users’ personalized needs with extremely low power consumption. It can effectively accomplish incremental learning tasks in edge computing scenarios, adapt to new scenarios and learn new knowledge with very low power consumption to meet users’ personalized needs.